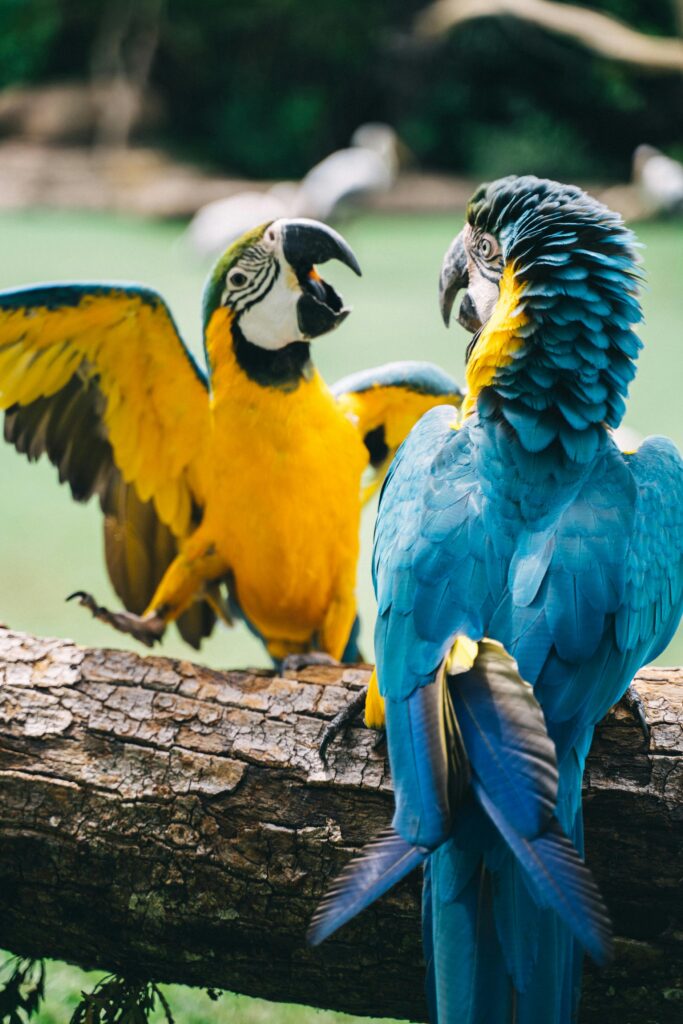
Photo by Anna Tarazevich
My experience is that almost any discussion involving Generative AI sparks passionate debate at the expense of informed discussion, knowledge sharing and consensus building. The internal algorithmic and cultural logic of social media platforms prioritizes the loud and adversarial in ways that can overwhelm important nuance even measured disagreement. This dynamic unhelpfully divides participants and even some observers into two opposing camps: those foresee mostly danger and catastrophe (“doomers”) and those who seem eager to embrace every new development and overlook risk (rather than “fanboys or fangirls” I will use “stans”). Within this framing there’s a variety of reasons stated for taking one side or another, “stans” are not necessarily blind, wilfully or otherwise, to the social, artistic, cultural, ecological, and economic concerns stated by so called “doomers”. Some apparently accept the march of technological progress, the good and the bad, as inevitable, definitely outside the control of the general public. No matter the rationale, a polarized framing presents a false dichotomy, one where the discourse is reduced to a tug-of-war between two extreme positions.
Leaving aside the fact that the term “AI” is at best, loosely defined and it’s not unfair to describe it as overburdened. It’s a sign signifying disparate technologies with a long and variegated history. The term sits in the public discourse with diverse applicability and implementations with computer scientists sometimes leaning into more precise terminology of ML, LLM, GPT etc. It’s unhelpful to view anything with applications as wide ranging with such rapidly evolving capabilities through a binary lens of either all good or all bad. As a category AI covers an expansive range of applications. It is used to identify or create life-saving innovations in healthcare and the wholesale generation of dangerous misinformation. Treating AI adoption in stark and simple terms is at the expense of nuanced realities that lie in between.
The risk of this are either the opportunity costs of not investing time, effort, and money in technologies that will define epochal change for the better or rushing into a disastrous consequences that could have been avoided. The contested histories of the Luddites and of the Environmental movement give us examples that we can reflect on when making a more thoughtful evaluation of AI and what we choose as a society to do with it.
Historical Lessons: Luddites and Environmental Concerns
It’s axiomatic that the Luddites were opposed to progress and wanted to preserve a way of life that was backwards in the face of technological advances. The facts are a bit more complicated, and the popular, mistaken version of those events has mostly to do with who got to tell the story in the end. “Freedom of the Press belongs to those who own one” as A.J. Liebling observed more than 60 years ago. More pointedly “history is written by the victors”. It doesn’t seem like many of Ned Ludd’s supporters even if they owned a printing press, they didn’t get to write the history most of us learned in school.
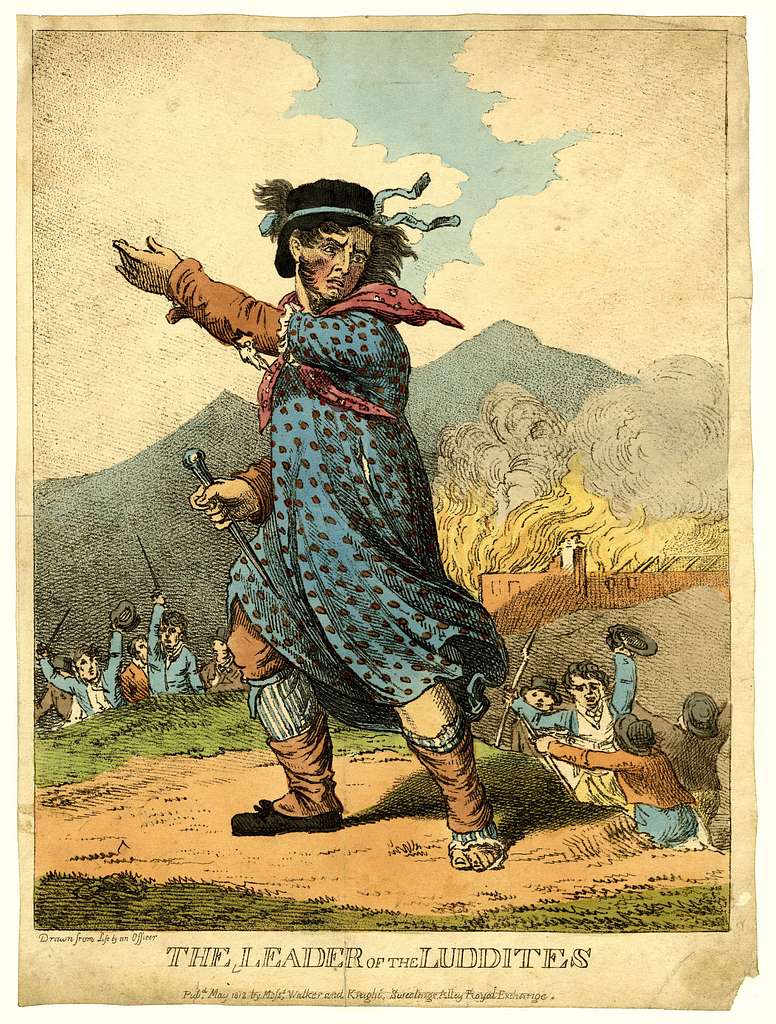
The Luddites: In the early 19th century, the Luddites—textile workers in England—became famous for their protests against the mechanization of their industry. Often depicted as anti-technology, the Luddites were not opposed to machinery itself. Rather their opposition was to the way it was being implemented, and how it would change the nature of work, and the life of workers. They feared that the new automated looms would not only displace their jobs but also degrade the quality of their work and lives. The Luddites ultimately lost their fight, their resistance highlighted the need for labor protections and served as an early example of workers’ resistance to owners unilateral actions. These set the stage for unionism and reformism that eventually led to the establishment of early labour protections. These laws have had lasting effects, such as the outlawing of child labor and the protection of workers’ rights, illustrating that even when resistance seems futile, it can clear the way for future reforms.
We can also learn lessons from the experience of how we’ve responded to the problem of climate change. There’s few and fewer people who are able to convince themselves or anyone else that we are not facing an epochal change in the climate. What some still find difficult to accept is that the damage and displacement, rising sea levels as well as unfathomable economic consequences were avoidable.
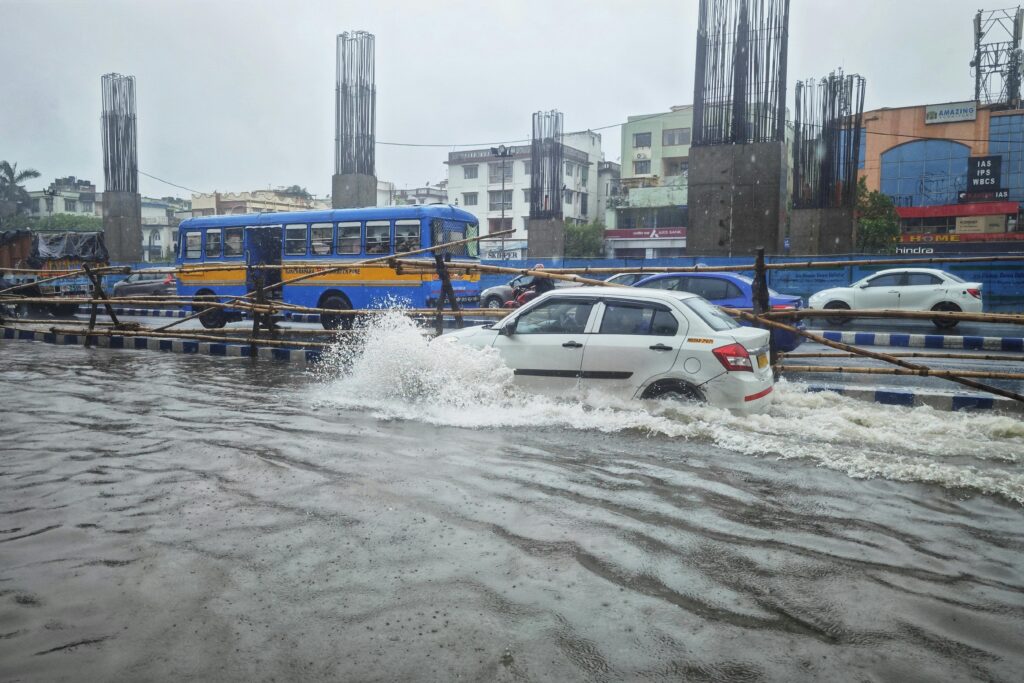
Photo: Dibakar Roy https://www.pexels.com/@dibakar-roy-2432543/
Climate Change: As early as the late 19th century, scientists expressed concerns about the potential dangers of burning fossil fuels and the greenhouse effect. However, their warnings were largely ignored, dismissed as alarmist or irrelevant. It wasn’t until the late 20th century that the gravity of climate change began to be acknowledged—and even then, resistance to addressing it continues now that we are well into the 21st Century. Significant changes to the climate are already underway, and we are now faced with the tasks of changing not only how we generate and distribute energy, we have the additional task of mitigating the impacts rapidly changing climate in ways that impact almost every aspect of our lives, flooding cities, drought in rural areas and extreme weather events everywhere. The current climate crisis and that it was mostly avoidable, had we chosen to act when the signs were already apparent, serve as to illustrate what can happen when valid concerns are dismissed in the name of enthusiastically embracing progress or growth in the short term, ignoring the medium and long term consequences.
These examples bracket modernity. The first is one of the defining cultural motif of the first industrial revolution in the English Speaking World. The second either optimistically signals events leading to a third, post carbon industrial revolution or pessimistically that societal collapse is on the horizon if we do not act decisively.
Together, they serve as reminders that skepticism and yes, even resistance need to be considered in how we choose to proceed. And collectively, as a society governed by laws and democratic institutions, we have the capacity to make these choices for ourselves and not accept Silicon Valley VC’s version of the March of Progress as inevitable and the only possible future.
People and organizations who are making the case that we should face the concerns surrounding the adoption of GAI—whether about job displacement, ethical implications, or environmental impact—are not simply “doomers.” They are raising important questions that must be addressed in order to adopt these technologies responsibly and sustainably. Nor are most so-called “stans” necessarily uninterested or dismissive of all the risks that adopting Gen AI involves.
AI’s Promises and Pitfalls
Cliché alert: “Generative artificial intelligence holds the promise of being one of the most transformative technologies of our time.” But it’s true: GenAI and AI generally are already changing medicine, research, and unburdening us of some of the uninspiring, Yak Shaving type tasks that seemed unavoidable even five years ago. However, alongside these promising developments come significant risks that need to be carefully considered.
The Promises: AI has already begun to deliver remarkable advancements across a wide range of fields. In medicine, AI-driven tools are improving diagnostic accuracy, enabling personalized treatment plans, and even aiding in the discovery of new drugs. In law, AI can streamline case research, automate routine tasks, and potentially make the justice system more efficient and accessible. Scientific research is being accelerated by AI’s ability to process and analyze vast amounts of data, leading to discoveries that would have taken decades using traditional methods. In software development, AI is assisting in code generation, bug detection, and the creation of more robust and scalable applications. Even in service industries, AI is enhancing customer service through intelligent chatbots and predictive analytics, leading to more personalized and efficient interactions.
The Pitfalls: Yet, these advancements are not without their downsides. One of the most concerning issues is the proliferation of poor-quality or even dangerously misleading AI-generated content. For example, imagine a generative AI system producing a guide on foraging for mushrooms—a task where misinformation could lead to fatal consequences. This highlights the broader issue of AI’s capacity to produce content that can be difficult to verify for accuracy, leading to potentially harmful outcomes.
Environmental Consequences: Another significant concern is the environmental impact of AI, particularly the immense energy and water consumption required to train and run large AI models. The process of training AI models involves massive computational power, which in turn demands a substantial amount of electricity. Data centers, where these computations are performed, are energy-intensive facilities, often powered by non-renewable energy sources. The carbon footprint of these operations is considerable, contributing to the broader issue of climate change.
Additionally, as AI models become more complex and widespread, their energy demands are escalating. A single large-scale AI model can consume as much energy as multiple households use in a year, and this energy usage is only expected to grow as AI becomes more entrenched in various industries. This not only raises concerns about the sustainability of AI but also about the opportunity costs—resources that could be allocated to other critical areas, such as renewable energy development, are instead being diverted to power AI technologies.
Water Usage: Beyond energy, the water usage associated with AI operations is another critical environmental issue. Data centers require substantial amounts of water to cool their servers and maintain optimal operating temperatures. As AI demands increase, so does the need for water, putting additional strain on water resources, particularly in regions already facing water scarcity. This diversion of water from agricultural, industrial, and domestic uses to support AI infrastructure can have far-reaching consequences, exacerbating existing environmental and social challenges.
For example, during periods of drought or in areas with limited water supplies, the competition for water between AI data centers and local communities or ecosystems can become a significant source of conflict. Moreover, the extraction and use of large quantities of water can disrupt local environments, affecting biodiversity and the availability of clean water for human consumption and agriculture.
The growing environmental footprint of AI underscores the importance of developing more sustainable technologies and practices. This includes investing in more energy-efficient AI models, utilizing renewable energy sources, and implementing water conservation measures in data centers. It also highlights the need for a broader conversation about the true cost of AI adoption and the importance of considering environmental sustainability alongside technological progress.
The Pitfalls of Bias in AI: One of the most insidious risks posed by AI is its potential to amplify existing societal biases, particularly against women, racialized minorities, and poor and working-class people. AI systems are trained on historical data, which often reflects the prejudices and inequalities embedded in our society. When these biased data sets are used to train AI, the resulting systems can perpetuate and even magnify these biases, leading to outcomes that disproportionately harm marginalized groups.
In hiring practices, AI-driven tools are increasingly used to screen resumes, assess candidate suitability, and even conduct preliminary interviews. While these tools promise efficiency and objectivity, they often inherit biases from the data they are trained on. For example, if a company’s past hiring data shows a preference for candidates from certain universities or with specific demographic characteristics, the AI system may inadvertently penalize candidates who do not fit that profile, perpetuating a cycle of exclusion. This can result in fewer opportunities for women, racialized minorities, and individuals from lower socio-economic backgrounds, further entrenching inequality in the workforce.
In medicine, AI has the potential to revolutionize healthcare by providing more accurate diagnoses and personalized treatments. However, medical AI systems trained on data that predominantly reflects white, male populations may be less accurate when applied to women and racialized minorities. This can lead to misdiagnoses, inadequate treatment, and poorer health outcomes for these groups. For instance, studies have shown that some AI systems used to predict patient outcomes may systematically underestimate the risk for Black patients compared to white patients, leading to disparities in care and treatment.
In law enforcement, AI is often used in predictive policing, facial recognition, and risk assessment algorithms. Unfortunately, these systems are prone to reinforcing existing biases in the criminal justice system. For example, predictive policing tools trained on biased crime data may disproportionately target minority communities, leading to over-policing and higher rates of arrest in these areas. Similarly, facial recognition technology has been shown to have higher error rates for women and people of color, increasing the risk of wrongful identification and unjust treatment. These biases not only undermine the fairness of the justice system but also contribute to the systemic oppression of marginalized groups.
The ethical implications of these biases are profound. They highlight the need for rigorous oversight, transparent methodologies, and continuous auditing of AI systems to ensure that they do not perpetuate discrimination or deepen existing inequalities. Addressing these issues requires a commitment to developing more inclusive data sets, implementing checks and balances, and engaging in ongoing dialogue about the ethical use of AI.
In short, while the benefits of AI are significant and transformative, the risks—especially the potential to amplify societal biases and its environmental impact—cannot be ignored. It’s essential to approach AI adoption with a balanced perspective, recognizing both its potential to drive progress and the serious challenges it presents.
Embrace Complexity
As the debate over artificial intelligence continues to evolve, it’s crucial to move beyond the simplistic “doomers versus stans” dichotomy and embrace a more nuanced understanding of AI. Both uncritical enthusiasm and unyielding skepticism can lead to flawed decisions and missed opportunities. Instead, what is needed is a balanced perspective that acknowledges the potential of AI while remaining vigilant about its risks.
AI has the power to drive significant advancements in fields ranging from medicine to scientific research, offering solutions to some of the most pressing challenges of our time. However, these benefits come with considerable trade-offs. The environmental costs of AI, including its substantial energy and water consumption, pose serious challenges that cannot be ignored. Moreover, the amplification of societal biases through AI systems risks perpetuating and even deepening existing inequalities, particularly for women, racialized minorities, and economically disadvantaged groups.
This balanced perspective doesn’t mean a tepid middle-ground approach; rather, it calls for informed, thoughtful engagement with AI. It means recognizing that skepticism about AI isn’t just fear-mongering—it’s a necessary check against unchecked technological expansion. Historical precedents, such as the resistance of the Luddites or the early warnings about fossil fuels, remind us that the concerns of today can lead to the reforms of tomorrow if they are taken seriously and addressed proactively.
For AI to live up to its promise, and avoid becoming seen by many or most as the Dark Satanic Mills of our generation, it must be developed and deployed responsibly. This requires a commitment to transparency, accountability, and to ethical standards that prioritize human well-being and environmental sustainability. It also demands continuous attention to and evolution of regulations and or well established professional practices that ensure that AI systems are fair, inclusive, and do not exacerbate existing social problems or become instrumental in the creation of new ones.
Let’s relegate the opposition of “doomers” against “stans,” to being an awkward reminder of how easy it still is to fall prey to the fallacies of false dichotomy. Or worse choose to leverage those fallacies in hope of staking out the high ground in some future ideological conflict. Instead, let’s foster constructive dialogue that brings together diverse, even divergent perspectives. That way together, we can navigate the complexities of AI adoption, ensuring that its benefits are maximized while its risks are carefully managed. This balanced approach is not only more realistic but also essential for shaping a future where AI serves the common good.