Note I wrote this post just before this happened and $1 Trillion in NASDAQ share value evaporated over the course of last Wednesday. A bad day for sure. I reckon there will likely be more of this to come.
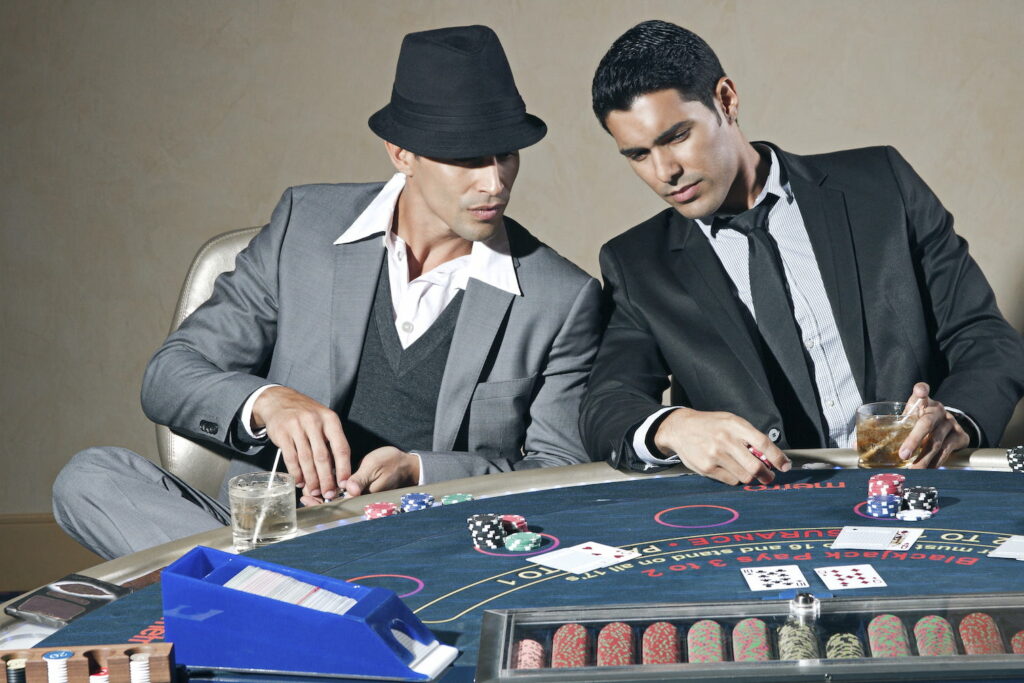
Are impatient investors cashing in their chips soon or letting it ride?
Observers agree there’s an AI bubble, but not as much when and how it pops
It’s not quite two years since the misleadingly named OpenAI launched ChatGPT 3 into the world spurring a virtual gold rush of the serious and unserious alike imagining infinite opportunities. Already there’s a consensus forming that it’s a bubble. Historically, bubbles burst.
The debate is around when and what will remain for the survivors to pick through in the smoking crater that is left after a bubble of this size bursts.
Tech giants have made impossibly large bets on a group of technologies that depending on who you ask, may never pay out
Goldman Sachs reports capital expenditures on AI by the hyperscalers: Microsoft, Apple, Google, Amazon1 will surpass 1 trillion dollars in the coming years. Optimistic observers suggest this time is different than before. Unlike the dot com implosion of 2000, companies have learned to keep am eye on ROI and won’t let a speculative bubble damage their bottom line.
In that same report Jim Covello Head of Global Equity Research at Goldman Sachs points out that “the substantial cost to develop and run AI technology means that AI applications must solve extremely complex and important problems for enterprises to earn an appropriate return on investment (ROI)”. He remains skeptical about the likelihood of that occurring within an acceptable time horizon for investors. He expects investor enthusiasm to begin to fade in 12-18 months.
Rather than a bursting bubble on the horizon, Covello exhibits guarded optimism with respect to continued the beneficiaries of AI development. Those are GPU makers (i.e. NVIDIA), power utilities and related industries which face increases in demand for electricity from data centres serving AI demand, and the hyperscalers themselves, who will experience incremental growth from the broader AI sector, and are well capitalized to navigate around any immediate problems.
Here, it is worth pausing for a moment, to clarify terminology. The phrase Artificial Intelligence (or just AI) gets used for a raft of different sometimes related sometimes unrelated technologies. In this context most of what we’re concerned with are the technologies related to the current state of Generative AI. Specifically Generative Pre-trained Transformers or GPT’s and LLM’s – Large Language Models. This is the category of AI that includes Chat GPT, Dall-E, Gemini, Grok, Co-Pilot etc. and includes the multi modal transformers used to generate (or synthesize) graphics, audio, and video as in addition to text. While these are related, indeed build on antecedent technologies like Machine Learning, Neural networks, Deep Learning we’re concerned with LLM and GPT here.
All the big, exciting uses for AI are either low-dollar (helping kids cheat on their homework, generating stock art for bottom-feeding publications) or high-stakes and fault-intolerant (self-driving cars, radiology, hiring, etc.).
– CORY DOCTOROW: WHAT KIND OF BUBBLE IS AI?
Way back in March of 2024 Richard Windsor posted a more pessimistic assessment of the same broad swath of data. He noted that there are Venture funded companies with valuations that are untethered from any fundamentals such as Cohere’s valuation of $5 billion on $13 million in revenue for 2023. Equally telling for readers of Windsor’s note, Microsoft’s hiring the CEO and 70 staff from Inflection AI, indicating a poor prospects for the AI Startup, because they went for it.
The assessments in Goldman Sachs report and Richard Windsor’s note, among others don’t seem to augur a cataclysmic collapse like the 2000 Dot Bomb implosion or the 2008 Global Financial Crisis. They do agree there will be casualties.
Cory Doctorow observed in an article for the January 2023 edition Locus Science Fiction magazine that “”All the big, exciting uses for AI are either low-dollar (helping kids cheat on their homework, generating stock art for bottom-feeding publications) or high-stakes and fault-intolerant (self-driving cars, radiology, hiring, etc.). “
The first casualties of the bubble popping, collapsing, or slowly deflating are most likely to be the low-dollar low risk cohort. They might get bought up by the bigger companies in the probably rare case where they have something uniquely valuable, consolidated or just disappear.
The collection of technologies under the AI umbrella have largely proven to be so far poorly suited for fault-intolerant work. Doctorow cites the example of an autonomous vehicle company Cruise. The General Motors Subsidiary was forced to take its vehicles off the road after series of reported incidents. Although as of May 2024 they have begun reintroducing their vehicles to public roadways.
There is not a lot of evidence to support claims that applying GenAI makes this kind of work faster, and more accurate. It’s difficult for me to see how they are going to mitigate the high stakes risks of their solutions without paying for skilled humans in the loop. The companies involved higher stakes-fault intolerant implementations will likely face the challenge of how much more expensive the AI assisted approach is relative to performing tasks like radiological analysis without them. Future analysis should evaluate the relative value or contribution of discriminative versus generative models. Discriminative models are a proven technology for tasks like classification or regression, sentiment analysis, and object detection. These are still the dominant models in use today.
[Anecdotally the impact of AI in the HR and recruiting systems has made them a galactic center where resumés of talented and experienced Designers, Project Managers and Software Engineers enter a Supermassive Black Hole without so much as emitting Hawking Radiation afterwards. Losing talent to a supermassive black hole seems likely to be expensive as well. -BB]
Ignoring Environmental Impact as the Earth is getting hotter
The first day of this week, July 21, 2024 was the hottest day globally since records of this kind were kept. It broke the record set 12 months earlier in 2023, and that shattered the record set in 2016. With that frequency of record breaking global temperatures, any remaining skepticism about anthropogenic climate change belongs well beyond the fringes of reasonable scientific or political discourse.
There will be no magic bullet to stem and eventually reverse the course we are on. There undoubtedly will be a mix of solutions applied with varying degrees of commitment, completeness and success. What isn’t in doubt is that any viable solution mix will involve electrification of some things that have historically involved locally burning fossil fuels. Things like electrified transportation systems, electric ground or air source heat pumps, even “smart” systems to tune industrial, commercial, and residential energy use are all going to make greater demands on the infrastructure needed to generate and distribute electricity. To say nothing of the already spiking demand in electrical power to cool buildings during increasingly long, hot summers.
No matter what the prospects for applications and implementations to justify the investment of dollars, time, and brain power in developing AI tools, there’s no question that LLMs consume a lot of energy. Power generation and distribution infrastructure improvements are time and capital intensive efforts. Even without the increased demand due to added computing power requirements, the electrification of transportation, home heating, and industrial uses could be either slowed or improved with data centres’ increased demand.
Cynically, I fear that when Microsoft, Amazon, Google, or Apple come knocking, utilities could prioritize the tech giants’ needs ahead of residential or commercial customers. Within certain limits around network latency and access to multiple data trunks, locating data centres is not as bound to geography as most of us are. Given that a company has optionality about where to locate a Data Centre, it can choose which utilities or jurisdiction is going to give the most favourable terms. Potentially this could pit the needs of an Amazon or Microsoft data centre against local residential or commercial needs. Given the need for consistency and reliability when powering a data centre, it’s likely to favour “reliable” fossil fuel power generation by default ahead of renewables.
Slowing the decarbonization of the economy to add technical capacity for technology that still promises more than it delivers seems bad enough. Although the companies involved in providing the cloud infrastructure for AI, tend to be unforthcoming with the details themselves, Melissa Heikkilä reported in the MIT Technology Review that estimates for training OpenAI’s GPT-3 and Meta’s OPT emitted more than 500 and 75 metric tons of carbon dioxide, respectively. Indeed Microsoft has all but thrown its hands in the air after making bold commitments in 2020 to be carbon neutral by 2030. Its greenhouse gas emissions were reportedly about 30 percent higher in fiscal year 2023.2 Similarly Google a company has made much in the past about its own commitments to carbon neutrality and sustainability, blew past its 2019 carbon emissions numbers by 50% in 2023. 3Its 13% year over year increase was apparently in large part to the increased energy demands associated with GenAI.
Is the juice really worth the squeeze?
or Who’s bluffing and who’s calling?
Being a prognosticator, fortune teller, or prophet is no easy job. So it should not surprise anyone there is no consensus to when the bubble bursts or what that burst looks like. Even where the AI boom is fueled by hyperbole and misdirection I suspect its collapse it won’t be as dramatic or convulsive as Enron, the 2008 Banking Collapse or the Dot Bomb. For the reasons outlined above I expect it to be more like a slow, sad deflation of expectations and investment capital. The bottlenecks of electricity supply and distribution as well as the chip supply chain (which is covered in detail in the Goldman Sachs document) are the more immediate technology threats to AI. Those, along with regulatory, and copyright infringement concerns may have consequences that will surprise many.
That the technologies clustered together as GenAI have consistently over-promised relative to what it’s delivered from some key observers’ perspective hasn’t seemed to slow the enthusiasm for investment yet.
Warning: I am neither a fortune teller nor a prognosticator, so don't consider this any kind of financial advice.
Last words: There is still no such thing as AI
Lastly, although this article is rife with the term AI, it’s grudgingly and arguably lazy of me. I have had problems with the term it since I first encountered it when a cousin of mine was working on the “AI” for SDI. For most technologists I know, anyway, AI is primarily a marketing term not a term of art.
To clarify: Most of what I have been writing about here is related to the technology of Large Language Models and Generative Pre-trained Transformers. These are sophisticated computational models based on the statistical relationships derived from evaluating the contents of vast amounts of text. It is unlikely that the ability to compute the statistical likelihood of what words will follow another represent any widely held concept of intelligence.
- Heh, a MAGA by any other name. Yeah seems Silicon Valley is heavily invested in Trump this year. Like Heidegger said about Naziism and technology, I guess. Or Walter Benjamin about Politics and Aesthetics, IDK. ↩︎
- Oh well, at least Bing’s hallucination bullshit can be entertaining as we collectively drive several fleets of deisel E-550s off the climate cliff. ↩︎
- Google hasn’t yet walked back its commitment to being “carbon neutral” by 2030 but it’s difficult to see how it will be possible given current trends ↩︎